Table Of Content
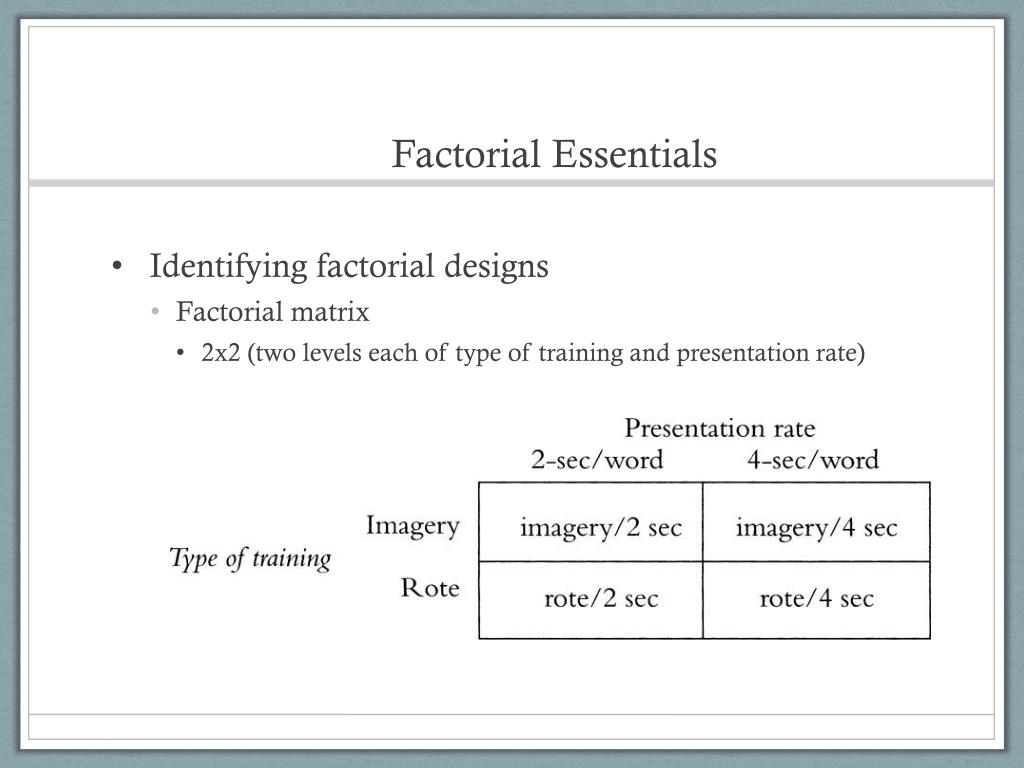
If they have poor internal consistency, then they should be treated as separate dependent variables. Manipulation checks are usually done at the end of the procedure to be sure that the effect of the manipulation lasted throughout the entire procedure and to avoid calling unnecessary attention to the manipulation. Manipulation checks become especially important when the manipulation of the independent variable turns out to have no effect on the dependent variable. Imagine, for example, that you exposed participants to happy or sad movie music—intending to put them in happy or sad moods—but you found that this had no effect on the number of happy or sad childhood events they recalled. This could be because being in a happy or sad mood has no effect on memories for childhood events.
5.1. Correlational Studies With Factorial Designs¶
If one of the independent variables had a third level (e.g., using a handheld cell phone, using a hands-free cell phone, and not using a cell phone), then it would be a 3 × 2 factorial design, and there would be six distinct conditions. Notice that the number of possible conditions is the product of the numbers of levels. A 2 × 2 factorial design has four conditions, a 3 × 2 factorial design has six conditions, a 4 × 5 factorial design would have 20 conditions, and so on. Also notice that each number in the notation represents one factor, one independent variable.
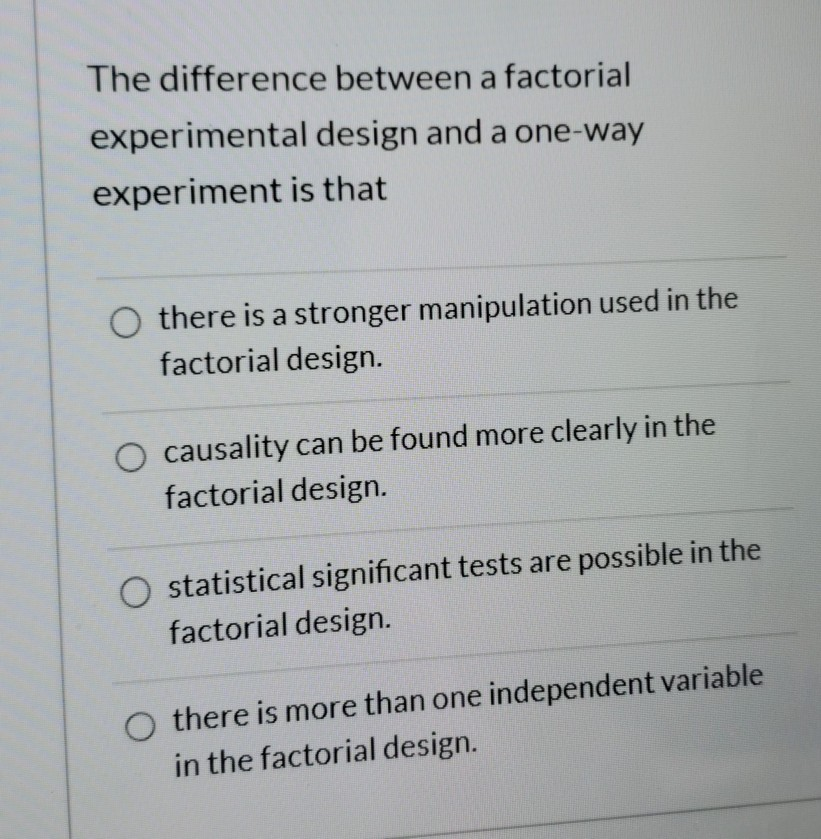
Implementing Clinical Research Using Factorial Designs: A Primer
Research like this, by the way, has shown both income and health make extremely small contributions to happiness except in the case of severe poverty or illness [Die00]. When we use a 2×2 factorial design, we often graph the means to gain a better understanding of the effects that the independent variables have on the dependent variable. An interaction effect occurs when the influence of an independent variable on a given dependent variable depends on the level of other factors being examined. It might be found, for instance, that the impact of salary on worker productivity may be more pronounced for entry level as opposed to experienced employees.
3 - Unreplicated \(2^k\) Factorial Designs
Through experimental design, researchers can test hypotheses and make inferences about the population from which the sample was drawn. When multiple dependent variables are different measures of the same construct - especially if they are measured on the same scale - researchers have the option of combining them into a single measure of that construct. Recall that Schnall and her colleagues were interested in the harshness of people’s moral judgments. To measure this construct, they presented their participants with seven different scenarios describing morally questionable behaviors and asked them to rate the moral acceptability of each one. Although the researchers could have treated each of the seven ratings as a separate dependent variable, these researchers combined them into a single dependent variable by computing their mean.
Author & Researcher services
An optimization method for multi-objective and multi-factor designing of a ceramic slurry: Combining orthogonal ... - ScienceDirect.com
An optimization method for multi-objective and multi-factor designing of a ceramic slurry: Combining orthogonal ....
Posted: Sat, 02 Jun 2018 21:49:55 GMT [source]
(In reality, there was no other participant.) Then they gave each participant 10 points (which could later be converted to money) to split with the “partner” in whatever way he or she decided. Because the participants were the “dictators,” they could even keep all 10 points for themselves if they wanted to. When researchers combine dependent variables in this way, they are treating them collectively as a multiple-response measure of a single construct. The advantage of this is that multiple-response measures are generally more reliable than single-response measures. However, it is important to make sure the individual dependent variables are correlated with each other by computing an internal consistency measure such as Cronbach’s \(\alpha\). If they are not correlated with each other, then it does not make sense to combine them into a measure of a single construct.
Lesson 5: Introduction to Factorial Designs
If more than one outcome variable is used in analyses, the number of models computed and effects tested grow quickly. Various approaches have been suggested for dealing with the challenge posed by so many statistical comparisons being afforded by complex factorial designs (Couper et al., 2005; Green, Liu, & O’Sullivan, 2002). As opposed to an RCT, where the focus is on demonstrating effects that are highly unlikely to be due to chance, the screening experiment is focused on relative promise of the tested ICs.
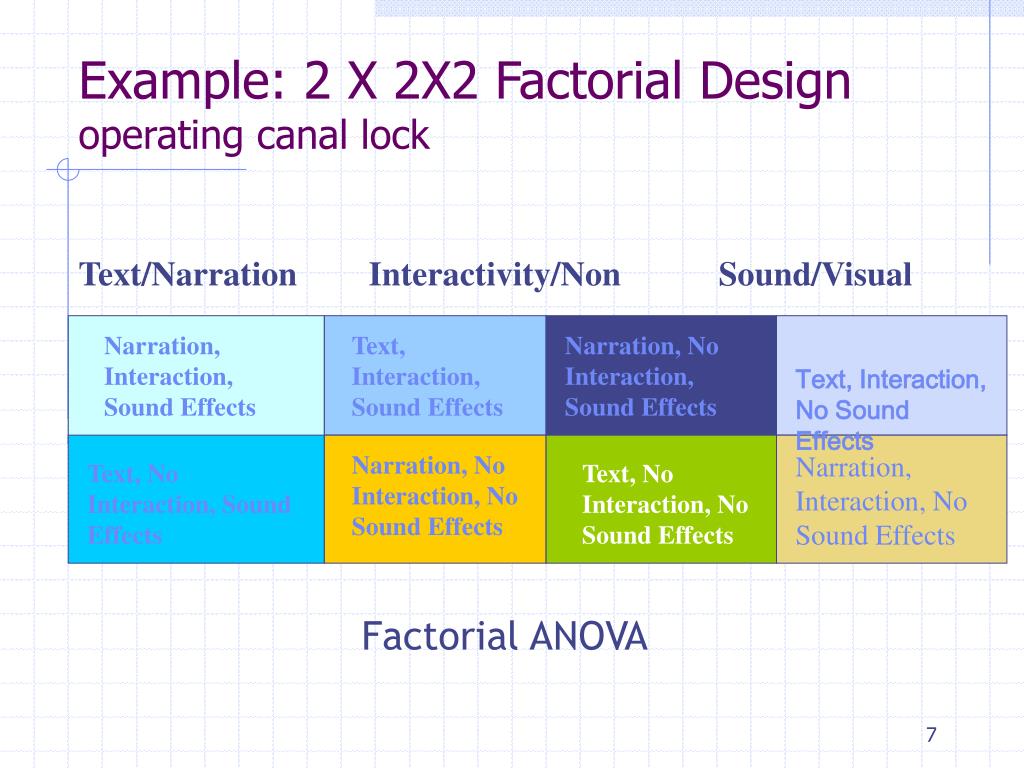
Consequently, this procedure offers a more efficient and cost-effective means to test several combinations of two or more conditions at the same time. However, the application of the design may prove more challenging as the number of levels and factors increases. One type predicts the main effects, which assess the influence of conditions across each factor separately.
But when we fit a reduced model, now there is inherent replication and this pattern becomes apparent. We will set this up the same way in Minitab and this time Minitab will show the plot in three dimensions, two variables at a time. When choosing the levels of your factors, we only have two options - low and high. You can pick your two levels low and high close together or you can pick them far apart.
A take-home message before we begin is that some independent variables (like shoes and hats) do not interact; however, there are many other independent variables that do. When the independent variable is a construct that can only be manipulated indirectly—such as emotions and other internal states—an additional measure of that independent variable is often included as a manipulation check. This is done to confirm that the independent variable was, in fact, successfully manipulated.
The second point is that factor analysis reveals only the underlying structure of the variables. It is up to researchers to interpret and label the factors and to explain the origin of that particular factor structure. For example, one reason that extraversion and the other Big Five operate as separate factors is that they appear to be controlled by different genes [PDMM08].
For information about the "Fold design" and "Add axial points", consult the "Help" menu. To have a total of 3 trials of each, the user should add 2 replicates in this menu. If 4 replicates are added, there will be a total of 5 trials of each.
You are given the following table that relates the combination of these factors and the students scores over the course of a semester. Use the Yates method in order to determine the effect each variable on the students performance in the course. Because experiments from the POD are time consuming, a half fraction design of 8 trial was used. Factors A - D can be renamed to represent the actual factors of the system.
No comments:
Post a Comment